Q&A with Members: Graeme Blair and Gwyneth McClendon
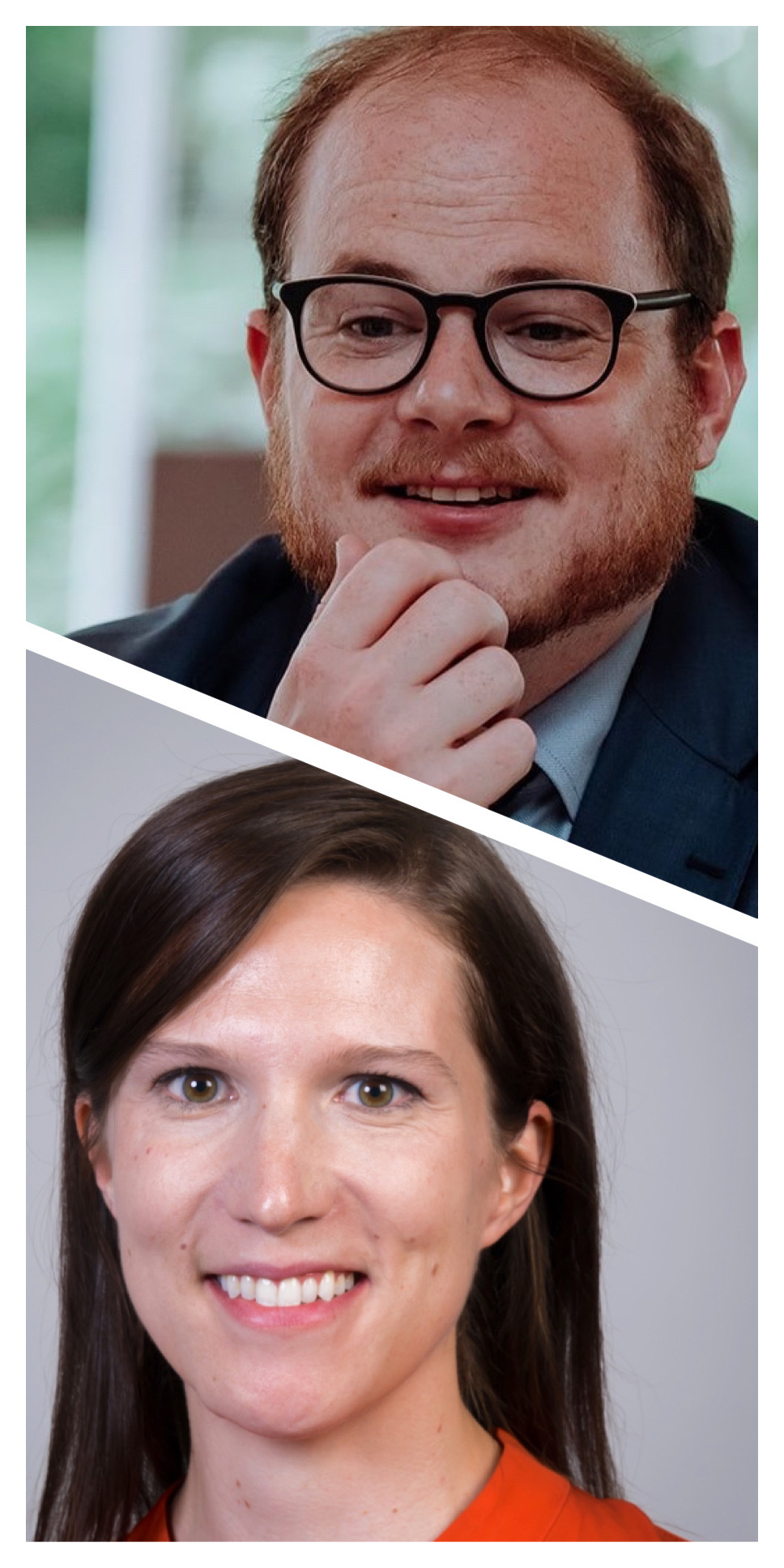
Authors: Jaclyn Leaver and Matthew Lisiecki
In today’s Q&A with Members we feature Graeme Blair, Assistant Professor of Political Science at UCLA, and Gwyneth McClendon, Associate Professor of Politics at NYU. We asked them about their recent chapter “Conducting Experiments in Multiple Contexts” in the forthcoming book Advances in Experimental Political Science, edited by James N. Druckman and Donald P. Green, and their reflections on what’s next for cumulative research programs.
Q: In your book chapter, you outline three types of cross-context experiments: uncoordinated, coordinated (sequential), and coordinated (simultaneous). In our recent blog post reflecting on the experiences of steering committee members who participated in the Metaketa Initiative rounds, we also outline three types of multi-site experiments: centralized, decentralized, and hybrid. Do you see room for a decentralized and centralized option within the two types of coordinated efforts described in the chapter? Or do you see coordinated cross-context experiments working only with a centralized administrative setup or only with a decentralized administrative setup?
Graeme Blair and Gwyneth McClendon: In our view, a centralized coordination body — like the Metaketa steering committees that oversaw and encouraged harmonization across multiple research teams — may be very valuable especially for simultaneous cross-country experiments. Coordination bodies can help solve a logistical problem and an incentives problem.
The logistics problem is that in conducting multiple coordinated studies at the same time, researchers not only need to share materials they develop — research designs, treatment protocols, survey questionnaires, and the like – they also need to align each element across studies, and they have to do so within a short period of time. In our experience, these tasks require much more work than a small team has time for in addition to designing and implementing their own study. A centralized coordination body can offload tasks that are repeated across studies and facilitate communication among teams to settle on shared protocols and measures.
The incentives problem is equally tricky but also aided by a centralized coordination body. The need to publish novel ideas and data, as the EGAP blog post notes, creates incentives that push scholars away from coordination. Compounding this incentive problem is that the research teams are making design decisions under uncertainty about what the results of the individual studies and the meta-study will be. The low-risk strategy is to adorn research designs with more and more treatment variations and more and more outcomes to be sure you have results that will be novel relative to the other studies. A centralized coordination body can serve as a countervailing force, advocating for harmonization (where appropriate), and facilitating conflict resolution where opposing ideas are proposed. Their power to do so may come from social pressure, the need for everyone to cooperate to achieve joint publication outputs, or if the coordination team helped fundraise for the studies and can withhold funding for noncompliance. In our experience, the pressure coming from both directions can lead to decisions that balance standardization with publishing incentives and that benefit the individual research teams.
When studies are happening more or less simultaneously, we think decentralized research would be logistically challenging and would be riskier for the researcher participants.
In sequential studies, the need for a centralized coordinating body seems somewhat less pressing, and the endeavor may be harder to sustain if it were centralized. On the one hand, the logistical problem of coordinating in a short time period is lifted. First-mover studies can post the materials for future scholars to adopt and adapt without the need for a centralized body. On the other hand, as we describe in our piece, these open science practices are still young and not always followed. Not all scholars share the details of research designs, treatment protocols, and materials, as well as data and code to integrate the actual results. Code and data sharing is now common in many social sciences; open materials and sharing research designs much less so. A centralized coordinating body could help to solve this issue for sequential cross-country experiments. However, because the centralized coordinating body would need to persist over time, serving as a member of a coordinating body for sequential cross-country experiments might constitute a more costly professional commitment and thus be more challenging to institutionalize. If the research studies themselves are conducted by the same lab in a highly centralized sequential research program, however, the professional benefits might outweigh these costs.
Q: In both your chapter and our blog post, the issue of researcher incentives is discussed. What are your suggestions for revising the incentive structure within each model that you outline to better align with incentives such as publication for career advancement, the costs and equity of access to funding, and networking opportunities for junior scholars?
GB and GM: In our view, each of the models we describe is flawed on these dimensions. The uncoordinated approach (“letting 1,000 flowers bloom,” as we term it) is already compatible with current publication incentives, which place a heavy emphasis on novelty and innovation. It does not, however, necessarily do a good job of addressing issues of equity or of opportunities for junior scholars, nor does it often facilitate meta-analyses for learning across contexts. The coordinated, sequential approach could be compatible with current publication incentives if one researcher or research team conducted all of the experiments over time, but this approach could fall prey to some of the vulnerabilities raised in the blog post about highly centralized research findings being less robust, and it would take significant (and concentrated) resources to carry out such a research program. The need for such resources could exacerbate inequities. And as we’ve mentioned the coordinated, simultaneous approach is also not terribly compatible with current publication incentives, though a centralized steering committee could provide networking opportunities and lobby for publication in the event of null results. Even still, the credit for the effort may still go disproportionately to the coordinating committee, and opportunities to be part of the effort could go to more privileged junior and senior scholars.
The bottom line as we see it is that (a) the field needs to deprioritize novelty relative to the incremental, cumulative findings that characterize most science; and (b) researchers or donors spearheading cross-country experimentation need to take steps not to reinforce typical privileges of opportunity and access. Deprioritizing novelty means donors’ targeting funds at replication in new contexts or rewarding proposals that incorporate past treatments or share outcome measures with previously conducted or ongoing studies (IPA’s advanced methods RFP is one great example of trying to fix these incentives). It means journal editors’ and reviewers’ giving favorable evaluations of research that seeks to replicate past findings or that shares interventions and measures with other studies. Taking steps not to reinforce existing privileges of opportunity and access means donors’ providing incentives for including local (often Global South) investigators on grants; it means widening the outreach for calls for proposals for coordinated experiments to include scholars at underfunded institutions (junior and senior) and from underrepresented groups; it means editors, reviewers and tenure committee rewarding research that incorporates diverse teams. These steps would likely also have the benefit of incorporating richer local knowledge that would facilitate the tailoring of treatments and outcomes to studies’ contexts.
Q: The chapter explains that it is most difficult to conduct a meta-analysis using uncoordinated studies because the participants, interventions, outcome measures and estimators all vary considerably across the projects. How do you make decisions about what to pool and analyze in an uncoordinated model without effectively going “fishing” for data that will provide you with significant results? What are best practices for making these decisions in the other models you outline?
GB and GM: Preregistration of meta-analyses is, we think, key. In medicine, it is standard to preregister the protocols for systematic reviews and meta-analyses, and the benefits would be similar in the social sciences. Preregistering both the targets of your search and a set of ex-ante rules for dealing with ambiguous cases would reduce the scope for fishing and alleviate readers’ suspicions. It is straightforward to identify the set of interventions of interest and outcomes that you suspect may have been collected without reading all the studies. Constructing a blank “gap map” would be one way to start (see 3ie’s Peacebuilding Gap Map for an example). Of course, there are aspects of preregistration that are more challenging for meta-analyses than for a single experimental study. Writing a pre-analysis plan for an experimental study can be done by simulating data as it will actually look when it is collected, using DeclareDesign for example. By imagining the data before it is collected, you can explore possible analysis plans blind to results. Imagining the data that result from a meta-analysis is more challenging: which intervention-outcome pairs will have sufficient data to analyze? The answer to this question is not always clear before you do a search (in fact, that’s one of the values of doing the search!); and given uneven adoption of data and code sharing and reporting of statistical analyses, which summary statistics will be available can be hard to predict. Increased sharing of research design details as well as data and code would make the process of pre-registering meta-analyses easier.
Q: Your chapter outlines four areas where social science is currently falling short in efforts to accumulate knowledge across contexts: how contexts are selected, how treatments are assigned to contexts, statistical power, and identifying not just what works but what works best in a given context. With inspiration from efforts to broadly facilitate meta-analyses of COVID-19 treatments, social scientists are beginning to develop platforms to encourage the generation of multi-site replications over time. These platforms mostly address the issue of data availability from previously-conducted studies. With the rise of these platforms, do you see the growth of coordinated, sequential research as something that is compatible with your call for addressing the four shortcomings described above? If you were involved in designing such a platform, what elements would you recommend for inclusion in order to encourage researchers to design their individual studies with those shortcomings in mind?
GB and GM: We applaud these efforts. We think coordinated, sequential research should become a standard part of the toolkit, along with uncoordinated research and Metaketa studies. We have three suggestions.
First, we think current data sharing practices for experiments do not go far enough: sharing open materials, research designs, data, and code should become standard. Authors could post treatment materials, such that later teams could replicate or adapt the intervention; measurement instruments, both in local languages and in English; and research design details about how units are sampled and treatments assigned, such as DeclareDesign code. Without these details, it is difficult to answer the same research question and contribute to the running meta-analysis or to identify new areas to add to the knowledge base by adapting or adding new treatments and outcomes.
Second, information on how areas and participants were sampled for inclusion in the study and how treatment effects vary across units should be collected in a standardized format. We identified the challenge of low power for making predictions across contexts because the focus has been on how effects vary across countries or cities in multi-site studies with a very small number of sites. Instead, we should take advantage of recent advances in treatment effect generalization, which require knowledge about how units were sampled and how treatment effects vary across units (see Egami and Hartman 2020). In addition to coordinating common outcomes and treatments, we should collect common individual-level and community-level characteristics that can be used to model treatment effect heterogeneity.
Finally, “gap maps” could be created from past studies along three dimensions: interventions, outcomes, and contexts. Among the challenges we identified were that: (1) treatment effects may vary by context and (2) existing cross-country experimentation does not involve a random sample of contexts but instead a set of contexts driven by an unknown set of selection drivers (that could be, among other things, convenience, and expectations of novel findings and so on). New coordinated, sequential research platforms would not overcome these challenges per se, but they could identify places where experiments on an intervention-outcome pair have not been conducted and thus incentivize researchers to fill that gap. The maps could be shared with funders, who could provide further incentives.